Thoughts by Gurinder Dua on a Linkedin post suggest an AI mission aimed at functioning as a digital public infrastructure (DPI) provider for startups adopts a centralised approach, framing “AI as a service.” Tanuj Bhojwani’s perspective opposes this, asserting that merely procuring 10,000 GPUs centrally won’t suffice. Bhojwani argues against the government adopting a purchaser or customer mindset for several reasons:
- Firstly, the rapid evolution of these technologies often leaves governments lagging behind, rather than leading innovation.
- Secondly, while centralised GPU mega centers are currently in vogue, the landscape has swiftly shifted. Just a few years ago, concepts like Transformers, fundamental to current LLM AI models, were discussed only among futurists.
- Thirdly, despite the emergence of centrally controlled models from tech giants like Google and OpenAI, the most rapid advancements are occurring in open architecture-based AI models such as Stability AI.
Moreover, future GPU demands might shift towards smaller AI models operating at the edges, away from centralised centers.
Furthermore, upcoming machine-to-machine architectures like those envisioned by cryptocurrencies such as Tensor or Helium could usher in a new era of decentralised AI, relying on DePIN (Decentralised Physical Infrastructure).
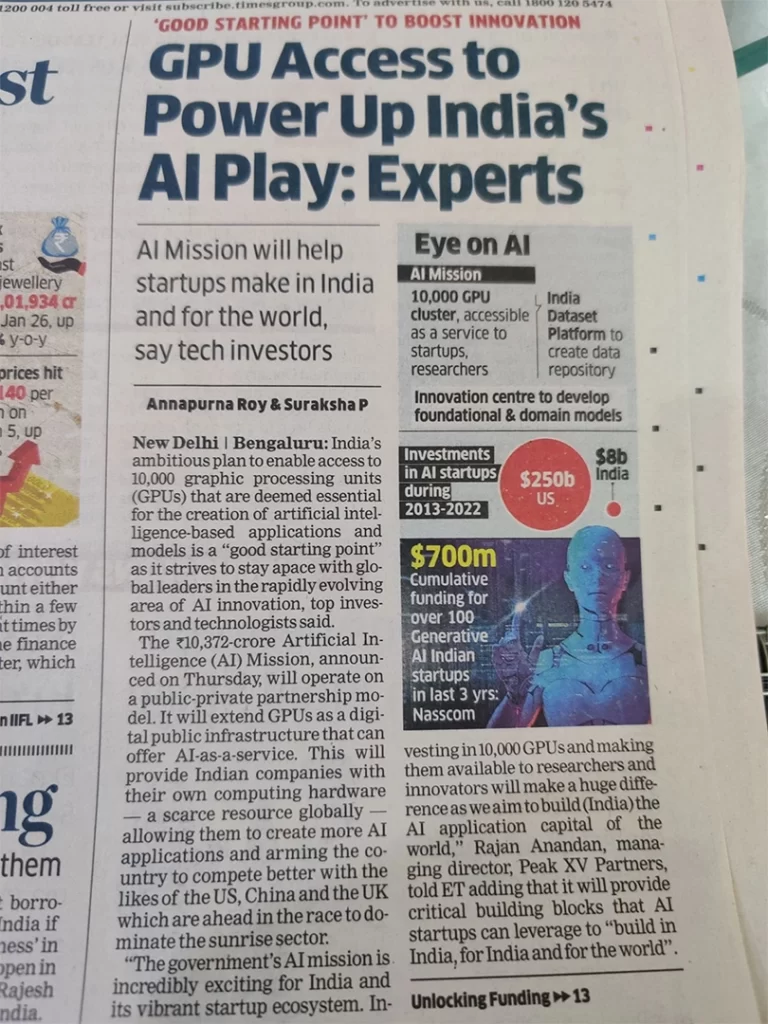
Public policymakers’ unfamiliarity with blockchain and cryptocurrencies suggests their hesitance to embrace the exponential changes anticipated with the convergence of AI, IoT, and blockchain technologies.
Innovation by dictate may not be the best approach for emerging technologies like AI, IoT, or even blockchain. However, there are alternative perspectives that shed light on the potential risks associated with centralised control in the AI landscape.
Gurinder Dua further emphasises the risks associated with the consolidation of AI power among major tech players. Dua suggests that the threat from centralisation of AI power may outweigh concerns related to political governance. Moreover, Dua advocates for decentralised approaches to AI development, exemplified by initiatives like the Bittensor protocol, as a means to mitigate these risks and foster innovation.